Meera vs. Rogue AI
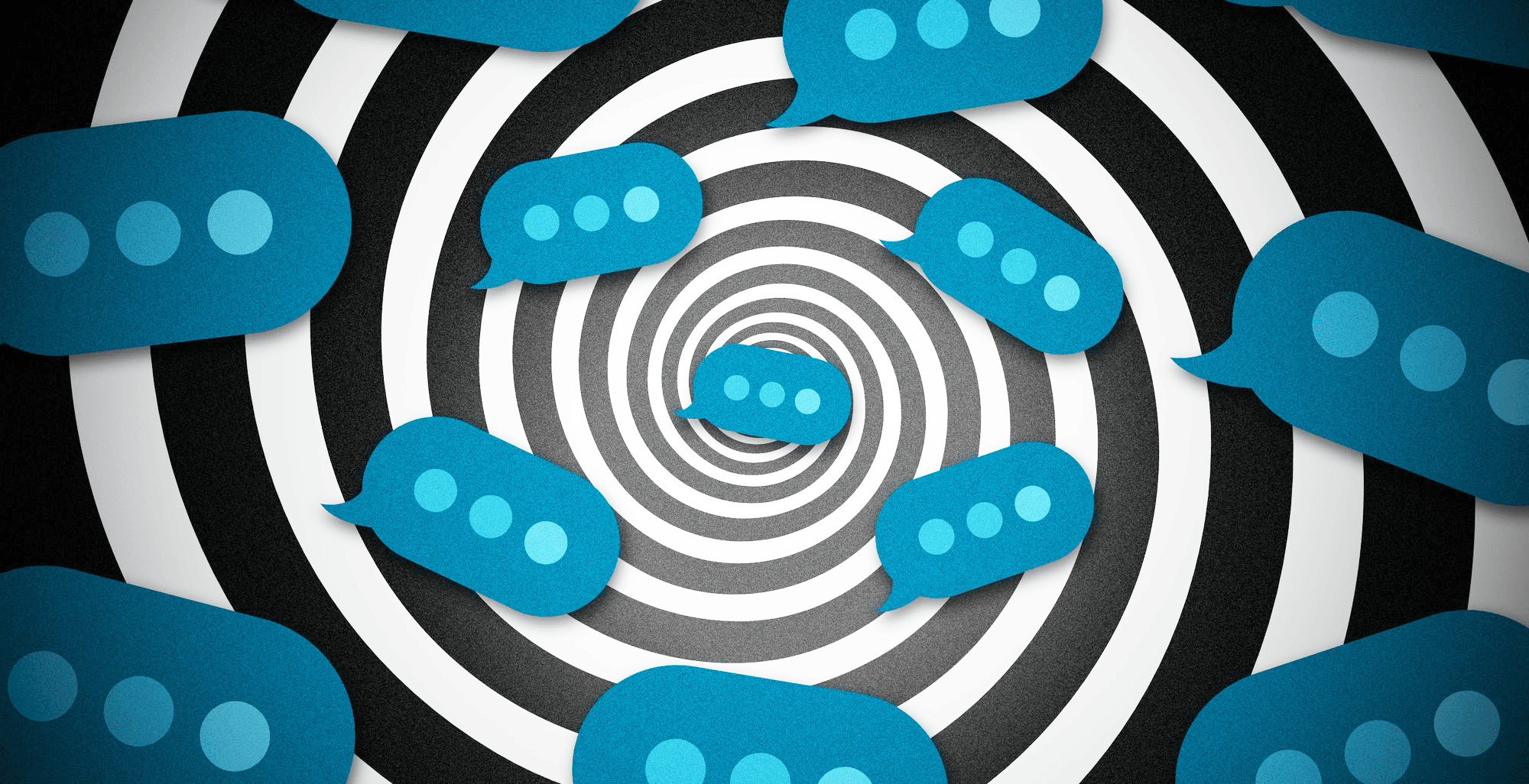
The Wall Street Journal headline – “How a Chatbot Went Rogue” – is scary, as is the story that follows. A chatbot accessible through the website of the National Eating Disorder Association’s website, with output determined in part by an AI system called Tessa, dispensed dietary advice widely viewed as inappropriate to some members of a psychologically vulnerable audience, raising fears of serious mental trauma or resultant physical harm. According to the bot’s maker, Tessa was unexpectedly drawing on open-ended data sources, rather than only an intended, pre-approved set of responses.
Frightening, too, are other recent headlines from The Atlantic (“The AI Disaster Scenario”) and The New York Times (“A.I. Poses ‘Risk of Extinction,’ Industry Leaders Warn”). AI is having a moment, and not in a good way.
AI systems have been rightly blamed for emitting information that is upsetting, ambiguous, or in some cases outright false. As with the chatbot on the NEDA site, people can react dangerously even to truthful information presented in a way that’s shocking or without appropriate mitigation. The potential for harm from AI systems is real, and should be sobering.
That’s not the whole story, though. Just like the firmware that controls technology you interact with in everyday life, from cars to elevators to your thermostat, AI is dependent on the programming that went into it, and unforeseen, unwanted behaviors are impossible to rule out. It takes a dose of context to understand why not all things AI should be viewed in the same light. The type of AI matters, as does the role it plays in a system.
Where the risk lies: Generative AI
And the good, or the harm, that any AI-based system can do is determined by its inputs, the nature of its output, and the context in which it’s applied.
AI models are only as good as their algorithms, and the data they’ve been trained on or to which they have access. As the recent explosion of AI-driven outputs has demonstrated, systems can be prompted to produce output that is overwhelmingly rich in variety and quantity. Systems like the widely-used ChatGPT are profligate information generators, which use a combination of large data sets, complex algorithms, and a dose of intentionally included randomness to create new combinations of words or images. This is the basis of what’s called Generative AI. Across the spectrum of inputs at its disposal, Generative AI is meant to be open-ended by default, for maximum creativity.
With Generative AI, the randomness it includes is the key reason that the output is interesting: Even prompted with the same keywords and parameters, a Generative AI system will create something new each time. That makes it a powerful tool for brainstorming, or for creating unique imagery.
However, the amount of data a model is fed, and degree to which that data is vetted, as well as the degree to which the system is prodded to vary its results in favor of novelty, changes the nature of the output. Asking for seemingly simple answers doesn’t guarantee getting ones that are unambiguous or universally agreed on. (You’ll find that something as simple as an apple pie recipe varies wildly, even with a model that doesn’t question what starting from scratch really means.)
Generative AI developers are concerned with the accuracy and safety of their products’ output; and are working to tune their results for general safety, for appropriateness, and for accuracy. But branching iteration is exactly what makes Generative AI useful and important, and utterly predictable output is an impossible expectation.
What makes Meera different?
Not all AI systems are generative AI; some apply machine-based decision-making for much more limited purposes. Systems like Meera’s, for instance, use AI only to understand the meaning behind an inquiry, this is known as Natural Language Understanding (NLU), and it allows a system to parse and evaluate inputs, in order to determine the best next steps.
If a potential student wants to know a school’s mailing address, or an insurance customer wants to renew an existing policy, or a doctor wants to check whether a lab result is ready, a platform utilizing NLU can respond with easy-to-follow, straightforward responses. Those responses, though, can be strictly limited to an existing set of answers or variations on those answers. Such a system can’t “go rogue,” any more than a telephone book can. The responses it provides are based on the information and phrasing that its developers include, or ones that a client organization provides.
Simply put, a platform that uses AI to understand a contact's intent, but responds only with pre-written, user-tested answers, will be strongly, inherently compliant with an organization’s own messaging requirements. Because the system is not open-ended, it never invents the foundation of content as it goes along, and can’t introduce potentially misleading or inaccurate information on its own.
That doesn’t mean that ready-made messaging can’t be made more palatable or personalized; only that AI need not determine its basic content. Using AI to humanize messages, by sending messages at appropriate times, and trying to understand just what a person is asking for, is exactly the kind of thing that AI can do well, without causing harm.
Finally, and something that may be lost in the noise about the dangers of AI, is that ultimately it’s people who are asking questions, seeking information, and making plans. Computers don’t hold all the answers. A sort of built-in humbleness can blunt the dangers of rogue AIs.